7 Data Science Applications for Healthcare Providers
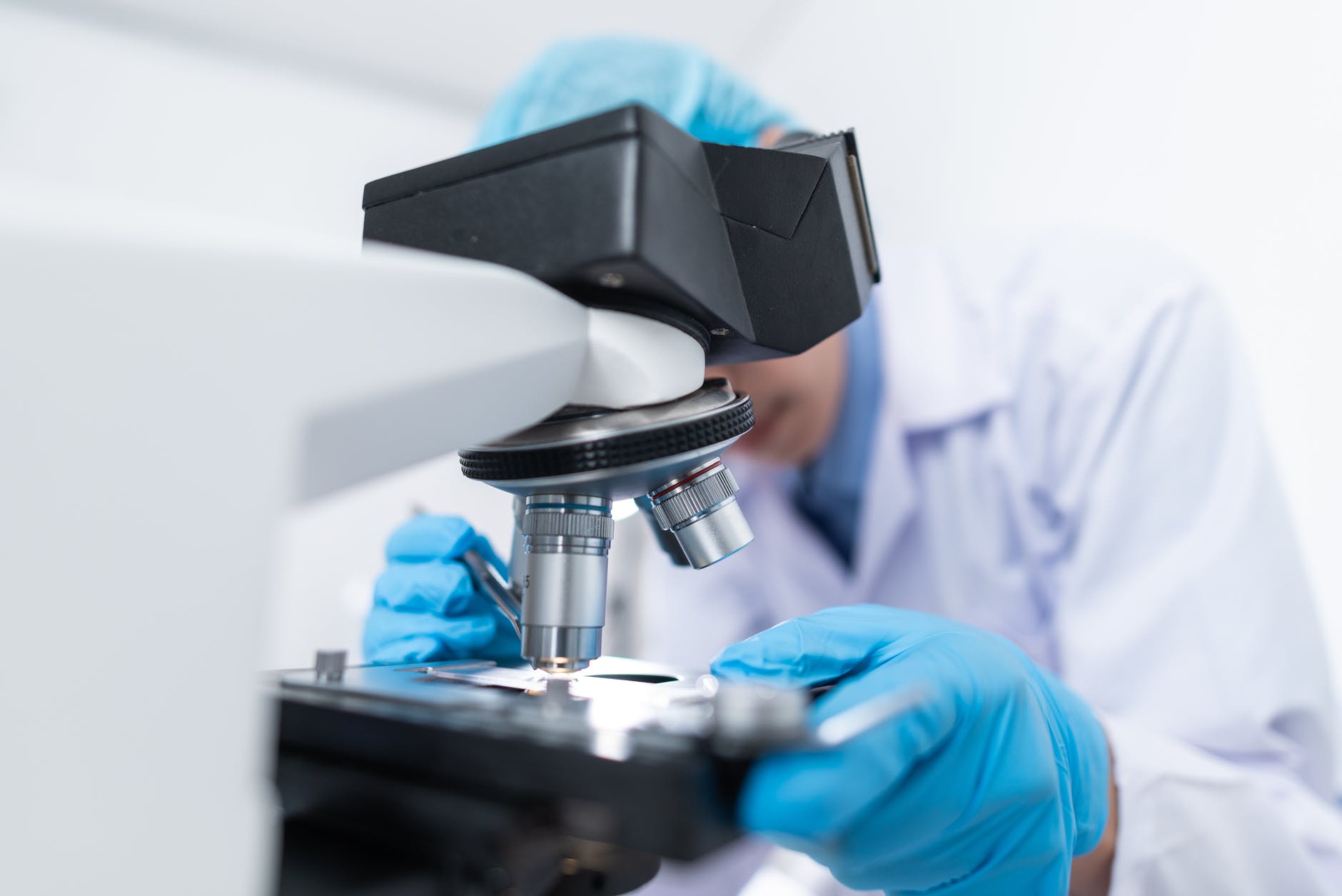
Data science has become an increasingly important part of healthcare. It’s used for everything from predicting which patients will develop diabetes to helping doctors diagnose cancer. Data scientists use algorithms to analyze large amounts of information to predict outcomes and guide treatment decisions.
Annually, 1.2 billion clinical reports are produced, and that’s just in the United States. Evidently, researchers could use a sea of health-related data to understand the human body, leading to the growth of informed healthcare.
Thanks to wearable techs, such as smartwatches, and even the iPhone in most people’s pockets, collecting data has become much easier. At the same time, there is difficulty in structuring and processing this vast data. Here’s where Data Science steps in and helps medical professionals process and interpret this data to make informed healthcare decisions.
The applications of data science in healthcare are vast; it has made it possible to detect the early signs of diseases and remotely monitor a patient’s condition. Hence, it has made it easier to increase access to healthcare and prevent diseases.
In healthcare, data science has helped advance the processing of data, including complex data sets retrieved from medical records, social media, genomic information, and information collected from wearable devices like smartwatches. It has helped improve analysis techniques by introducing artificial intelligence for systematic and unstructured data. In addition, it has also helped in the collection and processing of existing data from clinical trials, citizen science, and previous research.
Thus, with data science, the health care industry has been able to collect new and useful information and gain insights from existing data.
Table of Contents
Uses of Data Science in Disease Prevention
Several health issues are caused due to specific heat-related behaviour patterns or genetic predisposition. Health-tech companies are investing in the research and development of smart devices which can collect and analyse an individual’s medical records and family history to predict the risks of various diseases.
With further developments, these devices could help detect early symptoms, which could prevent the worsening of the individual’s health. Given that chronic health issues such as diabetes, hypertension, and more often have a genetic link, these devices could be revolutionary for disease prevention and early detection. In fact, data from such devices could also help remotely monitor a patient’s health, reducing the resources required to care for them. Hence, it would ultimately make extending care to more people easier.
Electronic Health Records collected using data analysis techniques have played a vital role in diagnosis and treatment. Using vast datasets, researchers have detected larger patterns in specific behaviour, symptoms, and diseases. Not only has this helped with early detection but also with developing patient-geared treatment as well.
Predicting Patient Outcomes
One of the first uses of data science was to help predict patient outcomes. This type of analysis helps doctors make better treatment decisions by identifying patterns in medical records. For example, doctors might find that certain medications work well with some diseases while others do not. They also might identify which treatments are more effective at treating different types of cancers.
Data science has been a boon, especially predictive analytics, to help detect, track, and monitor diseases. In the US, approximately 25 million people suffer from Asthma, and the numbers have been rising consistently since the 1980s. Asthma is a chronic condition, and it can be quite expensive. In 2007 alone, it cost about $56 billion in healthcare costs. In addition, the condition is unique and can be exacerbated by short-term exposures to triggers in the environment as well. Hence, a predictive analysis could play a vital role in keeping asthma attacks at bay by predicting likely triggers. While acute triggers of asthma have been identified with years of research, it is important to determine the fine-scale triggers. Over the years, research into the triggers has mainly relied on public data collected across the state, county, and even city level to ensure accurate predictions.
Unfortunately, most of the data were outdated or had gaps, as people would traditionally record symptoms and triggers in personal notes or diaries.
The data collection problem was easily resolved with advanced technologies, such as the Asthmapolis sensors that could be attached to inhalers. The sensor would passively capture the GPS coordinates, location, and time every time the inhaler was used. Then, a smartphone application would sync with the sensor, collecting and storing the data and allowing users to enter further detailed information such as perceived triggers, the symptoms they experience, and what they were doing when they needed to use the inhaler.
Then, this data would be uploaded to a remote server in real-time, and detailed analytics would be provided to the user and their caregiver. Analytics would include details on medication adherence and events that exacerbated the condition and provide an opportunity to create a data feedback loop to ensure better adherence to the treatment.
Within four months of using the Asthmapolis sensors, users experience better control and awareness of their asthma symptoms.
Predictive Analytics in Healthcare
Approximately 20% of the population suffers from autoimmune diseases in the US. These 80-100 diseases cost $100 billion, which is likely an understated estimate. Health-related costs have always been a point of contention in the US economy, reaching an astonishing $4.1 trillion in 2020. As the cost of treating autoimmune diseases, among others, keeps rising, healthcare-related costs will also increase.
But, rising costs often prevent access to healthcare, so how should they be addressed?
The answer seems to be data science or predictive analysis.
With healthcare analytics, medical professionals have been able to leverage useful insights from data, which has helped with accurate diagnosis and optimal treatment plans. In addition, data scientists help in processing and validating existing and new health-related data, which helps medical professionals make better diagnoses. As data science in the healthcare field grows, there is an immense opportunity for both predictive and prescriptive analysis to reduce costs related to monitoring diseases.
Data Science plays a vital role in predictive analysis in the healthcare industry. With the COVID-19 pandemic, the concern for public health has increased.
For example, during the coronavirus outbreak, the Centers for Disease Control and Prevention (CDC) analysed data from hospitals and clinics to predict the next possible outbreak and understand the severity of the disease. In the healthcare industry, predictive analysis helps in understanding the future course of a disease and helps guide decisions and policies within the healthcare industry.
Monitoring Diseases with Data Science
With multiple data sets, it is much easier to track symptoms and monitor a patient’s health as well. As with the example of the Asthmapolis sensor, it could help in predicting flare-ups or tracking whether asthma symptoms have gotten better or worse.
One of the biggest obstacles to effective treatment is patient adherence. Often, patients tend not to adhere to their medication, which could be for various reasons such as cost barriers, unpleasant side effects, or difficulty in obtaining specific medication. Unfortunately, treatment becomes much more expensive when patients do not or are unable to adhere to medication.
With data analytics, it can be easier to monitor patient adherence to treatment plans and allow medical professionals to engage the patient or alter the treatment plan to suit their situation. It facilitates better communication, but it ensures that the treatment plan proceeds as planned and ultimately helps save healthcare costs.
Apart from monitoring individual patients, but also the spread of infectious diseases. Interestingly, in the case of Covid-19, medical professionals may not always have to rely on health-related data to predict a new outbreak of the disease. For example, people observed that a spike in bad reviews for Yankees candles typically reviews ranging from “wrong smells” to “no smell at all,” corresponded to spikes in Covid-19 cases across the US.
Analytics used for healthcare is not limited to health-related issues. Though some may receive more attention than others because of their severity, the best part about data analytic models and machine learning is that they can be used for many diseases. Hence, with the increased adoption of data analytics across healthcare, the overall costs related to healthcare could be reduced, making it accessible to more people.
Improving Medical Imaging with Data Science
The advent of deep learning technologies such as artificial intelligence and machine learning has also helped medical imaging. Though several medical imaging techniques such as X-Ray, CT scan and MRIs exist, these images need to be manually inspected. Unfortunately, even though these images help visualise the inner parts of the body, microscopic irregularities are challenging to detect manually, which prevents proper diagnosis.
It’s not just the analysis of images that have benefited, but image enhancement, reconstruction, and recognition have been made easier with Data Science. Hadoop, a Big Data platform, has enabled medical professionals to use a tool called MapReduce, which can obtain parameters for several tasks, such as lung tissue planning.
Streamlining Drug Discovery
Drug discovery is a complicated, time-consuming, and expensive process. Generally, it can take up to 12 years and about $2.5 billion to research and develop life-saving drugs. Naturally, pharmaceutical companies strive to streamline this process and gain quicker approval from the FDA (Food and Drug Administration), which would help cure patients faster. Hence, data science shows immense promise in this field.
For example, BenevolentAI, a leading example of a clinical and pharmaceutical development company with fully integrated AI, aims to develop models and algorithms, such as a bioscience machine brain which could help advance discovering capabilities in this field. With data science, pharmaceutical companies can lower costs, reduce failure rates, and increase the pace of medicine delivery. Researchers could develop general models using machine learning technology and test different combinations of drugs against specific skin cells and genetic mutations. Thus, it could ultimately be a life-saving technology used for drug discovery.
Accelerating Diagnosis and Treatment
With advancements in medical imaging, data science has already advanced the ease of diagnosing various diseases. However, analysis of specific patterns in data could help detect chronic diseases as well. For example, a data analytics algorithm model is faster at detecting irregular heart rhythms by analysing ECGs than even experienced cardiologists.
Even in treatment, data science does not fall far behind. As more specific patient-related health information is made widely available, it is much easier to detect patterns and abnormalities. It is much easier to detect genetic links and plan a treatment course without the use of traditional drugs.
Virtual assistants, such as Ada, created by a Belin-based start-up, can also help in predicting diseases by analysing a user’s symptoms. Additionally, companies such as ScienceSoft are developing analytics software which could be a boon in the health care industry. Such analytics could help bridge the patient and doctor communication gap, helping track patient symptoms and treatment plans. Using a mobile application, patients would enter their current treatment plan and symptoms and check whether introducing a new medication could hinder their existing treatment.
Listing the various ways in which data science could be, and already has been, applied within the healthcare industry is not easy. There is no other industry where data could play such an important role than the healthcare industry; with data science techniques, healthcare industry revolutions would become faster, aiding treatment and saving lives.
Data Science Aids Disease and Symptom Detection
In the US, billions of health-related data are recorded every year. With big data sets, it is easier to make population-level inferences and personalize them as well. With the help of data scientists, big data allows medical professionals to make discoveries or determine personalized risk factors, for example. For genetic or hereditary diseases, early detection is key. Hence, by introducing other variables into the mix and existing health-related data, it may be easier to understand an individual’s susceptibility to various diseases.
Identifying personal level risk factors makes it much easier to provide people with effective treatment plans and how they can manage or prevent their disease. Thus, it facilitates better doctor-patient communication and makes disease-related information more effective as it is specifically geared toward them rather than being a generalized recommendation. For example, specific dietary nutrients are useful for some but harmful for others when it comes to nutrients. With massive data sets and statistical techniques, it is much easier to determine whether an individual is likely to experience beneficial effects from a nutrient.
Evolution of Data Science in Mental Health
In 2001, William S. Cleveland coined the term “data science” to explain data analysts’ contributions to the scientific field, suggesting that there is room to incorporate data science in research to streamline the process. Understanding data is crucial in any field; after all, almost everything has to do with data.
Data science refers to collecting, processing, visualisation, analyses, preservation, and re-using data or extensive information collection. “Big data”, a term often used when discussing data science, refers to a large amount of data that is often difficult to process using traditional data analysis methods. Hence, it necessitates the use of high-speed and real-time data analyses techniques.
As referenced earlier, Data Science isn’t restricted to one field; where there’s data, there’s data science. So, it makes sense that Big Data has gained prominence in the field of mental health as well.
Role of Big Data in Mental Health
When used in Psychology, data science could help open up new doors; in fact, it has already helped streamline data collection and analyses, which has helped enhance research practices. The use of data analyses and its tools can drive research in unexplored domains, such as computational neuroscience. As Gomez-Martin et al. point out, it could help develop “ethomes”, referring to detailed descriptions of behaviours across species.
However, it is essential to note that “big data” is nothing new to the field of Psychology; the discipline is built on data. Of course, how data was collected has changed over the years, and luckily, has become far more manageable than before. From the 1880s where psychologists like Hall would use questionnaires to collect descriptive data to the scales used by Likert and Thurstone, which helped render data more amenable to statistical procedures, big data has been around for quite some time.
Today, software like IBM’s SPSS or other open-source alternatives have made it much easier for psychologists to manage the data they deal with. With such software, relevant areas from data science can be used to easily address key issues in psychology. They not only help with data visualisation but also with large-scale collection of data along with exploratory and confirmatory data analysis. Advancements in data science have, thus, not only made it easier than ever to compute and interpret data but helped researchers collect and deal with vast data more effectively.
Use cases of data science in Psychology
Thanks to the benefits of predictive analysis, data science has already had a significant impact on psychology. Traditionally, statistical techniques such as multiple regressions would be used when developing a predictive model. However, thanks to machine learning tools in data science, predictive analysis was much more manageable, allowing psychologists to explore non-linear relationships between independent and dependent variables, no matter how large the data may be.
Data science has various use cases in the field of psychology. In their book “Big data at work: The data science revolution and organisational psychology,” Tonidandel, King, and Cortina (2015) highlight the importance of data science in industrial and organisational psychology. After all, companies need to analyse and interpret data and understand what it means in terms of human behaviour. Unlike mathematicians and statisticians, industrial psychologists have the unique skill to interpret human behaviour.
Thus, when combined with data science, it could save companies from making million-dollar mistakes. For example, organisations struggling to retain employees need to invest more in recruitment, which ends up costing a pretty penny. With the help of data science, industrial psychologists can answer questions such as “What makes employees stay longer in a job?” or “What type of employees are more committed to the goals of the organisation?”. These questions don’t merely satiate curiosity, but help organisations guide their decisions, especially regarding their human resource strategies.
Markowetz (2014) and Fawcett (2016) have also pointed out the collection and use of data for clinical research, especially those referred to as “quantified self”. Fawcett points out that people collect data on themselves thanks to tech innovations such as Fitbit or one’s iPhone. Researchers point out that such big datasets could be beneficial in healthcare and wellness, where analysis could help establish stronger links between health, productivity, and individual behaviour.
Take hypothesis testing, for example, its importance in the field of experimental psychology has been highlighted again and again, especially in the context of data analysis. About 90% of the research in this field draws conclusions on the basis of hypothesis testing, and the use of data science has made it easier. Now, researchers can parse large data sets much more easily. For example, a 2017 study by Frison and Eggermont sought to understand whether a reciprocal relationship existed between different types of Instagram uses and Adolescents’ depressed moods. Using data science tools, the researchers successfully analysed over 600 responses. The study concluded that both increased browsing and posting on Instagram were related to increases in adolescents’ depressed mood, and this relationship remained the same for both girls and boys.
Ultimately, using a data science approach in any field leads to more accurate and efficient use of data. At the same time, it’s much faster, even when large data sets are involved. When used collaboratively in psychology, data science helps give meaning to non-psychological data as well. For example, medical data collected from hospitals could provide valuable insights into the effectiveness of health interventions, especially individual behaviour.
Future expectations
Data science is a boon to the scientific field, from helping researchers assess the efficiency of new medicines to allowing practitioners to test theories on cognitive behaviors. As both healthcare and data science evolve and intersect, the possibilities for human well-being will become endless. Healthcare analytics is set to play a major role in the overall well-being of humans.
References
https://link.springer.com/book/10.1007/978-3-030-76732-7
http://www.philippe-fournier-viger.com/books/ai_disease_book/index.php
https://www.liebertpub.com/doi/pdf/10.1089/big.2013.0027
https://towardsdatascience.com/using-data-analytics-to-predict-detect-and-monitor-chronic-autoimmune-diseases-ca062e9cb12c
https://www.liebertpub.com/doi/10.1089/big.2013.0027
https://www.ncbi.nlm.nih.gov/pmc/articles/PMC7123557/
https://www.cdc.gov/coronavirus/2019-ncov/science/about-epidemiology/monitoring-and-tracking.html
http://psychlearningcurve.org/a-case-for-data-science-in-psychology/
https://www.oxfordbibliographies.com/view/document/obo-9780199828340/obo-9780199828340-0259.xml
https://www.researchgate.net/publication/339525231_Data_Science_Methods_for_Psychology
https://www.jstor.org/stable/10.5406/amerjpsyc.131.4.0477
https://datasciencedegree.wisconsin.edu/blog/history-of-data-science/